views
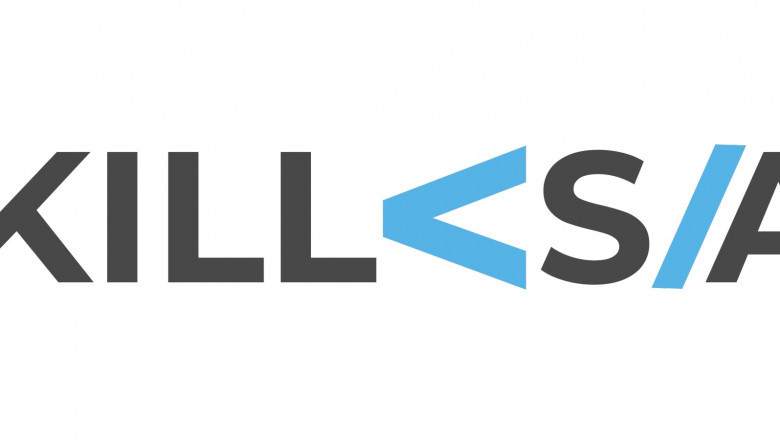
You must have heard the terms "Artificial Intelligence", "Machine Learning", and "Deep Learning" quite often, and that too interchangeably. However, for all those who aim to make a ground-breaking career in AI, it's imperative that you understand that all three are different.
Lexico defines AI as computer system theories and developments that perform activities that would otherwise (and usually) require human intelligence. Activities include but aren't limited to decision making, visual perception, and speech recognition. While you might have heard AI computer systems are smart, they cannot learn on their own. This hole is filled by machine learning and deep learning, and in today's article, we'll understand the difference between these two highly important concepts.
Machine Learning: Introduction
A type and subset of artificial intelligence, machine learning is a field where ML algorithms enable software applications to become more precise and accurate without the need to program them to do so. With the advent of machine learning, we witness present-day computer systems learn from the inputted data without the need to be continually reprogrammed. In simple terms, these systems constantly enhance their performance for any given task or activity. For example, when playing a chess game, you can choose a different level against the CPU (easy, moderate, hard, very hard). Here the system improves its performance without any human intervention or help.
One can leverage ML in various fields such as Finance, Healthcare, Art, and more. For educational purposes, there are different ways through which we can get machines to learn. Two prominent ways include:
· Basic Decision Tree
· Artificial Neural Networks
Deep Learning: Introduction
Deep learning is basically a subset of both machine learning and artificial intelligence. It’s a field that concerns itself with imitating the ways how humans gain knowledge. Experts consider DL an important aspect of data science that includes statistics and predictive modeling.Various well-known individuals and companies state (through experience) that deep learning will turn out to be machine learning's next frontier, i.e., the cutting edge of the cutting edge. The possibilities are you've already experienced the outcome of a deep learning algorithm even without knowing or realizing it.
If you have a Netflix subscription, I assume you've already viewed the recommendations it poses to you as to what you should watch. But, not just that, you might have experienced a streaming-music service that selects a song based on what your choices and selections were in the past and for which music you have hit the like or thumbs-up button.
Both the examples discussed are based on DL. However, a more prominent display of deep learning usage is Google's voice and image recognition algorithms that leverage deep learning. Just like ML is known as an AI type or a subset, the same is said for deep learning, i.e., it's a type (or a subset) of machine learning.
Machine Learning vs. Deep Learning: 5 Points of Differentiation to Understand
Human Involvement or Intervention
In the case of a machine learning system, human involvement is required, where an individual identifies and hand-codes the laid characteristics based on the data type. Whereas a deep learning system automatically tries to understand and learn those features, not requiring any human intervention or involvement. Let's take help from a facial recognition software example to understand this better. Here, the program first understands and learns how to detect and recognize face lines and edges and then hops onto other important parts of the face. Finally, it goes to the overall face representation. We must understand that the data usage in this is enormous. With the passage of time, as the program trains itself, the possibility of getting more accurate and correct recognition increases by notches.
Leveraging Hardware
Deep learning leverages a more robust hardware when compared to simpler ML systems. The reason for the same lies in the fact that a mammoth amount of data is getting processed and the mathematical calculation complexities concerned in the algorithms leveraged. A prominent example of hardware leveraged by deep learning is a GPU (graphical processing unit). On the other hand, machine learning systems run on low-end machines without requiring as much computing power.
Time Consumption or Requirement
By now, you have understood that in the case of deep learning, there are massive data sets as requirements, and there are various parameters and intricate mathematical formulas involved. Therefore, a DL system will require a lot of time for training purposes (a few hours to a few weeks). On the other hand, time consumption by machine learning systems ranges from a few seconds to a few hours.
Method or Approach Followed
In the case of deep learning, the systems view an entire problem in one fell swoop. On the contrary, in the case of machine learning, the systems parse data into parts, which are then combined to receive a solution or the result. Let's understand this with an example. Let's suppose you want a program to identify a certain object in an image, say a license plate on a car parked in the parking lot. Taking ML into consideration, you would require to undergo two steps: object detection and recognition. However, with DL, you just need to input the image, and with effective training, the program returns the identified object and its location in the image in one result.
Various Applications
It's believed that if you've gone through the differences mentioned above, you now understand that both these concepts have different applications.
Machine Learning
· Predictive programs (forecast stock market price)
· Email spam identifiers
· Evidence-based treatment plans for better medication solutions for patients
Deep Learning
Apart from the examples discussed in the earlier points (Netflix, music-streaming service, facial recognition), self-driving cars are another great example of deep learning systems. Here, the system leverages various neural network layers to perform actions such as determining objects to avoid, identifying traffic lights, and, most importantly, understanding when to speed up or slow down.
Final Words
Machine Learning and Deep Learning are the latest hot topics, and they are here to revolutionize customer experience, business automation, and how we live our lives. If you are an aspirant wishing to get into any of these domains, there's no time better than today. Being recognized as the best data science institute in Bangalore, Skillslash provides the best data science course with placement guarantee. With live classes led by industry leaders, be sure to learn these concepts in detail, and secure a high-paying job. Contact Skillslash’s support team to know more.
For More Information Visit :- https://skillslash.com/data-science-course-in-bangalore/